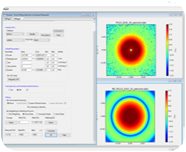
Example of 2D model-fitting using the SasView application | 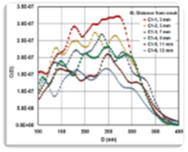
Cavity size distributions in a steel weldment as derived from SANS
10.1179/1743284714Y.0000000577
| 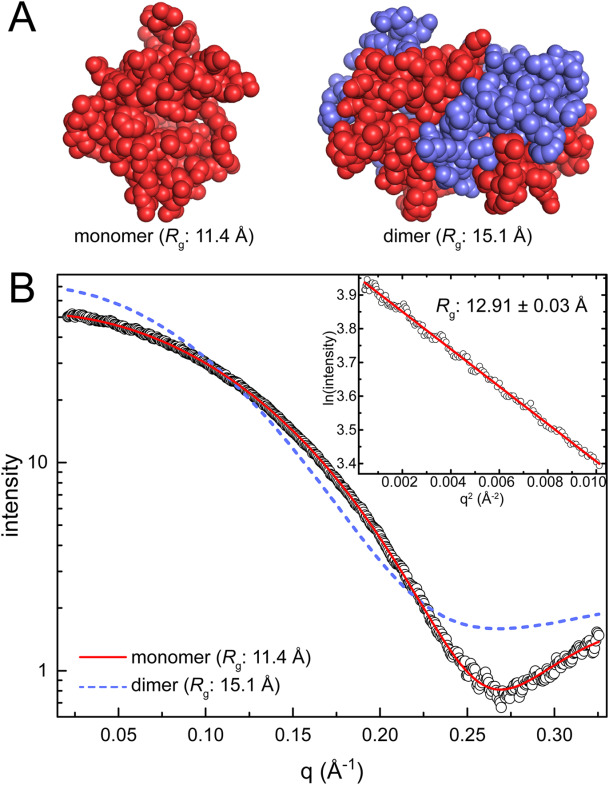
Ab-initio modelling of polcalcin constrained by SAXS
10.1002/pro.3376 | 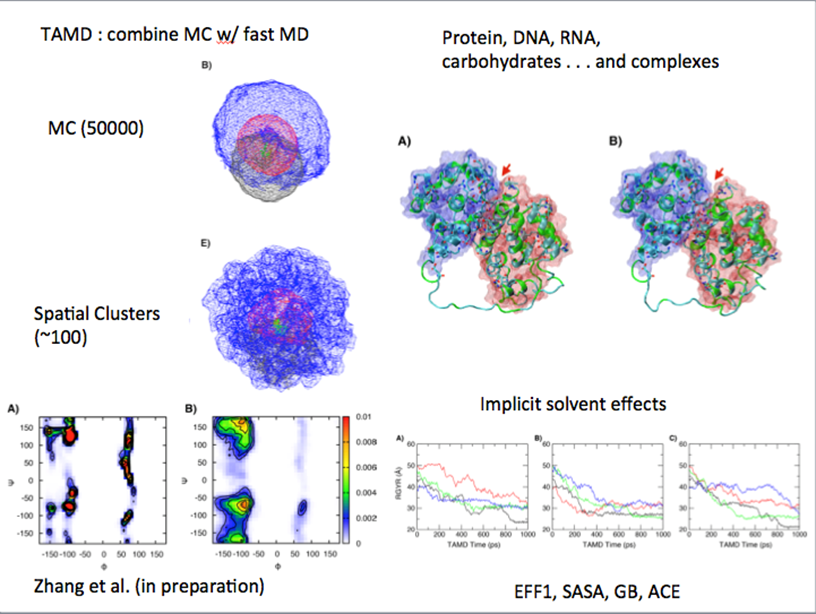
MC & TAMD modelling of proteins constrained by SANS
10.1016/j.jmgm.2017.02.010 | Time evolution of the invariant during crystallisation of P4MP1
10.1038/pj.2012.204
|
This approach uses iterative optimisation to match the calculated scattering from a model function describing the scattering objects to the measured scattering data. Each iteration one or more physical parameters describing the model (e.g. concentration, size, scattering length density) are adjusted.
| This approach uses mathematical transformations (e.g. Fourier Transforms) to convert the measured scattering data in reciprocal-space (i.e. in Q-space) into a function in real-space. Typical outputs are density correlation functions, volume fraction distributions, and size distributions.
| This approach uses iterative optimisation to match the calculated scattering from assemblies of spheres or from a 3D 'shape envelope function' to the measured scattering data. Each iteration the number and/or position of the spheres, or the curvature of the envelope function, is adjusted.
| This approach uses iterative optimisation in combination with Monte Carlo (MC) and/or Molecular Dynamics (MD) techniques or RRT searches to match a calculated 'atomistic level' structure for the scattering objects to the measured scattering data.
| Other approaches to data analysis may involve identifying, for example: any Q-dependencies in the measured data, particular patterns in the Q-values of any peaks present, asymptotic extrapolations, calculation of the integral under the measured data (the 'invariant'), or the intensity at Q=0.
|
This approach:
- is easy to learn;
- is good for well-defined scattering objects (e.g. nanoparticles, micelles, vesicles, polymer coils, etc) or combinations of these;
- works best if some a priori information about the scattering objects is available (e.g. shape, approximate size, etc) to guide initial parameter values;
- is generally quick, especially for 1D data (i.e. I(Q) vs Q);
- can work on 2D data (i.e. I(Qx,Qy) vs Qx & Qy);
- can allow co-optimisation of data of different contrasts (or even of SAXS and SANS data);
- can allow for magnetic-SANS;
|
This approach:
- is typically used to analyse data from (semi-)crystalline polymers, adsorbed polymers, voids/pores in solid materials, or nucleating systems;
- is model-independent;
- needs high-quality data (i.e. good signal-to-noise) to transform;
- needs data over a wide-Q-range (at least 3 decades);
|
This approach:
- requires an investment in learning to use it well;
- is well developed for solution structural biology, but has been used to simulate micelles, etc;
- does not require a starting structure;
- does not require any force fields;
- ignores any chemistry and physics (there are no chemical bonds, for example);
- can allow for hydration layers;
|
This approach:
- requires an investment in learning to use it well;
- is presently really only developed for solution structural biology, though a goal is to extend it to more generic soft matter systems;
- requires an 'atomistic' starting structure (e.g. from the PDB or a MD trajectory);
- requires suitable force fields;
- is compute-intensive (though there are some cloud-based implementations);
- preserves the chemistry and physics of the structure;
- can allow for hydration layers;
|
This approach can provide:
- fractal dimensions;
- the type of ordering (fcc, bcc, hcp, etc) in the sample;
- the specific surface area of the sample (S/V);
- persistence lengths;
- osmotic compressibilities;
|
Software packages for this include:
- SasView
- SASfit
- Scatter - Irena - WillItFit |
Software packages for this include:
- (G)IFT*
- Corfunc*
- MAXE
- PRINSAS**
- McSAS
*included in SasView; **obsolete |
Software packages for this include:
- ATSAS Suite
- FoXS
-Shape2SAS
|
Software packages for this include:
- SASSIE (incl. SCT)*
- MultiFoXS - Shapespyer
*also see CCP-SAS
|
Software packages for this include:
- various (incl. spreadsheets) |